Amazon Fresh has seen a sharp spike in demand since 2019. During that time, the service expanded to more cities and became free for Prime members. Amazon Fresh also expanded its selection to include tens of thousands of products, including a complete grocery selection, everyday essentials, gifts, and more and also introduced one- and two-hour delivery.
In 2020, customer excitement for Amazon Fresh was fueled by the introduction of Amazon Fresh grocery stores. Amazon Fresh stores offer innovations like the Amazon Dash cart, allowing customers to shop by skipping the checkout line, features like Order Ahead on the Amazon App, and the ability to interact with Alexa at various locations throughout the store.
“Whether offline or online, we are constantly improving the grocery shopping experience for customers,” says Wei Gao, Amazon vice president grocery, product, and supply chain. “We are leveraging decades of Amazon operational experience and scientific innovation to develop thoughtful approaches that make grocery shopping faster and more convenient.”
From forecasting to selection, Amazon Fresh scientists are working through (often open) research challenges to develop machine learning models that seek to enhance the grocery shopping experience.
Inventory management done right
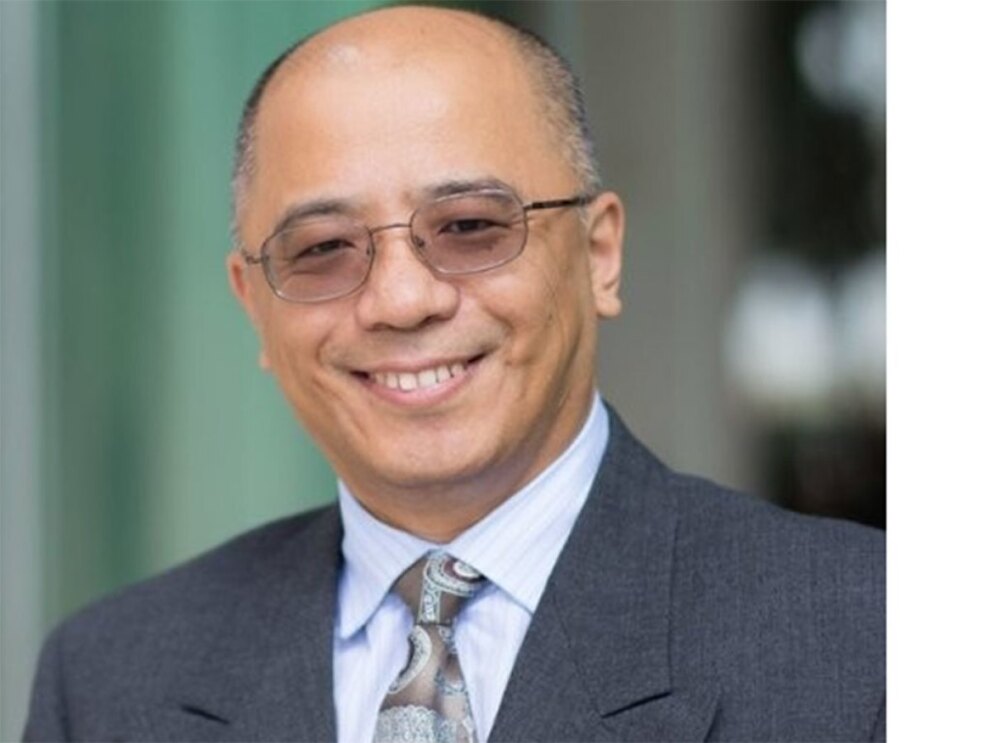
Tommy Jiang is the director of product and technology for Amazon Fresh.
Across Amazon, forecasting models help estimate future demand to help retail businesses decide which products to order and where to store them. Jiang’s team builds on this work to leverage data science and help Amazon Fresh make purchasing decisions. As part of this mandate, the team develops forecasting models — critical in grocery, where products have a limited shelf life.
“The localized nature of groceries can make forecasting a challenge,” says Jiang. “A customer order for strawberries in Seattle cannot be fulfilled from a store in New York. You can’t solve this problem by having everything everywhere. That would be wasteful.”
Amazon Fresh’s suppliers include manufacturers, distributors, and local farmers. To have high in-stock selection while also ensuring minimum waste, Jiang’s team must ensure that they are buying products from suppliers with the most relevant selection and optimal delivery times.
Scientists on the forecasting team have developed time series models to enable Amazon Fresh’s vendor-selection system to arrive at purchasing decisions in an automated way. The algorithm seeks to continually help Amazon Fresh customers access the best quality products at the lowest possible price.
“We are working with two sets of variables,” says Jiang. “Our models are always trying to determine what the demand for a particular product will be in a particular marketplace at a certain moment in time. Our models allow us to determine which vendor to buy a product from while taking factors like quality, shipping times, and price into account.”
Jiang’s team leverages Amazon’s Elastic Map Reduce (EMR) cloud-based service to process large amounts of data from multiple inputs on the supply and demand side. Amazon EMR offers built-in machine learning tools, including Apache Spark MLlib, TensorFlow, and Apache MXNet to create and customize predictive analytics toolsets.
Forecasting models developed by Jiang’s team helped Amazon Fresh improve inventory availability by 15% after the outbreak of COVID-19. While Amazon Fresh, like other grocery chains, was affected by the nationwide disruption to the grocery supply chain, scientific innovation helped alleviate some of the inventory concerns.
“Ultimately, the challenge of working through so many constraints — be they related to shelf life, inventory availability, or tight delivery windows — to drive improvements at scale is what makes Amazon Fresh an exciting place for a scientist,” says Jiang.
Product selection and more
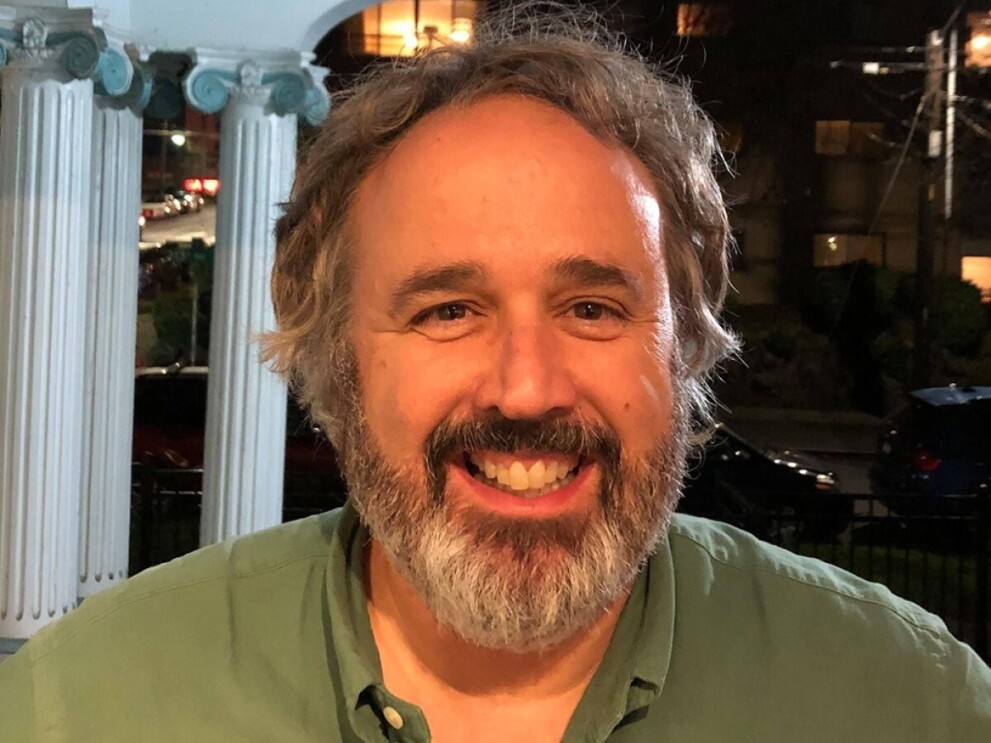
Gus Lopez is director of technology for Amazon Fresh. His team focuses on forecasting, and another area where scientists are playing an increased role: product selection. Think of the last time you walked through a cereal aisle at a supermarket. There are more permutations of breakfast cereal than you can possibly imagine — and then a little more. Lopez’s team uses signals like customer demand for a product, consumption data, and other factors to inform the ideal assortment of products.
Coming up with the ideal selection in the world of grocery is complicated for another reason. Consumers shop for groceries differently than they do for most items on Amazon. A shopper looking for the latest Stephen King novel might not settle for a novel from another author. However, when it comes to grocery shopping, consumers are more likely to opt for substitutions — for example, a shopper might be willing to buy a different brand if their favorite is out of stock.
For Amazon Fresh, decisions related to substitutions are driven by a machine learning model to generate pairings between different products. The model assigns a rank to each pairing using human opinion as a source of training data. This approach allows Amazon Fresh to analyze the rankings between different pairings, and recommend the most apt substitutions.
Just like any other team at Amazon, we are taking a customer-centric approach to this challenge.
Machine learning also helps with pricing anomaly detection, by automatically detecting mismatches between key product attributes and correcting them. For example, machine learning models can help differentiate between a can of soda as compared to a six pack for the same brand, and also identify mismatches between product images and titles.
Scientific innovation, Lopez notes, will continue to play an important role at Amazon Fresh across the entire customer lifecycle.
“Just like any other team at Amazon, we are taking a customer-centric approach to this challenge,” says Gao. “We want to anticipate our customers’ needs, so that grocery shopping is simple and convenient. The work of our scientists is helping us achieve that mission."